"The LLM and GenAI space has become incredibly non-transparent with costs getting out of control. Unify is the one-stop-shop for quickly comparing all of the latest models and providers objectively."

Lasse Espeholt
Staff Research Scientist,
deepmind
"When deploying new AI features for the Awin platform, which serves over 30,000 brands and 250,000 active partners, model diversity, evaluation benchmarks and performance optimization are vital. There is a constant stream of new LLMs with new capabilities every week, each becoming increasingly specialized. Routing across the entire LLM landscape could result in much higher quality than any one model, and Unify is therefore perfectly positioned to create a lot of value."

James Bentley
AI and Strategy Director, Awin
"Agentic RAG systems often involve dozens of sequential LLM calls, making speed and cost evermore important. Unify is perfectly positioned to address this, by intelligently routing to faster and cheaper models where possible. If they get things right, they could become an integral part of the LLM stack."
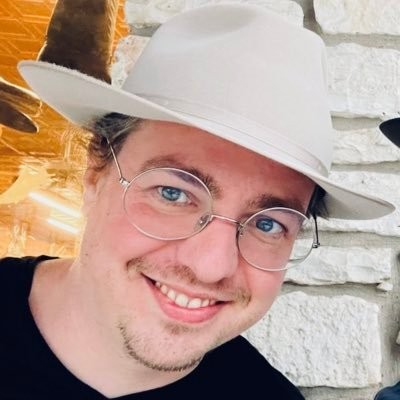
Bob van Luijt
CEO, weaviate
"Every new LLM claims to have the best performance on benchmarks, and every new provider claims to be the fastest and cheapest. Unify puts these claims to the test in an unbiased manner, on a central platform. Don’t take the models and providers at their word, just train a Unify router on your own data and see for yourself which combination is best."
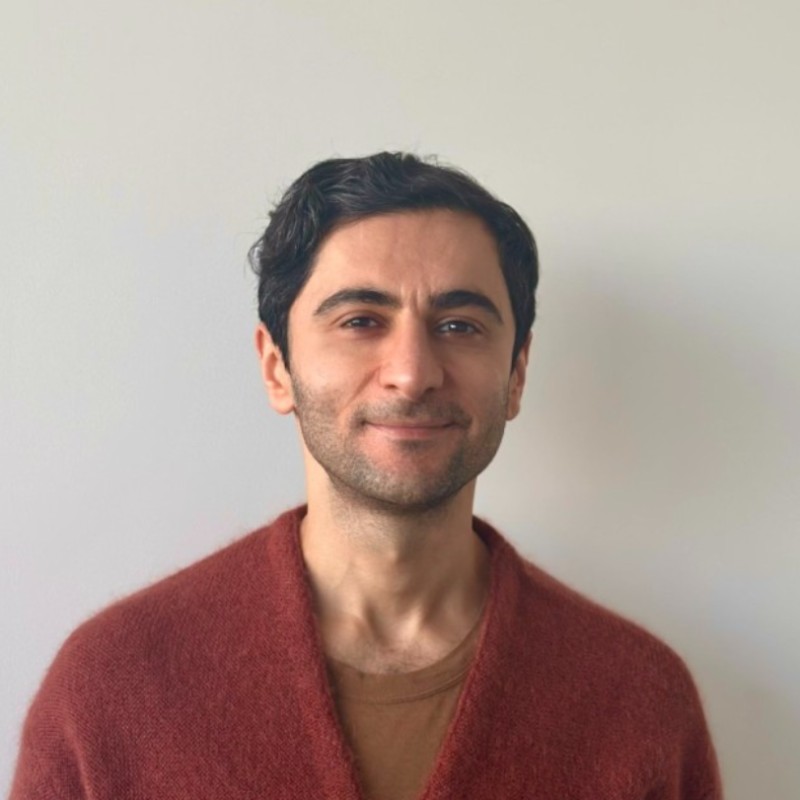
Arash Ferdowsi
Co-founder, dropbox
"As the LLM landscape continues to expand, solid and impartial developer tools are needed to ensure everyone is able to build high-performance applications. Unify is an integral part of this stack, giving a lot of flexibility to developers with little overheads."
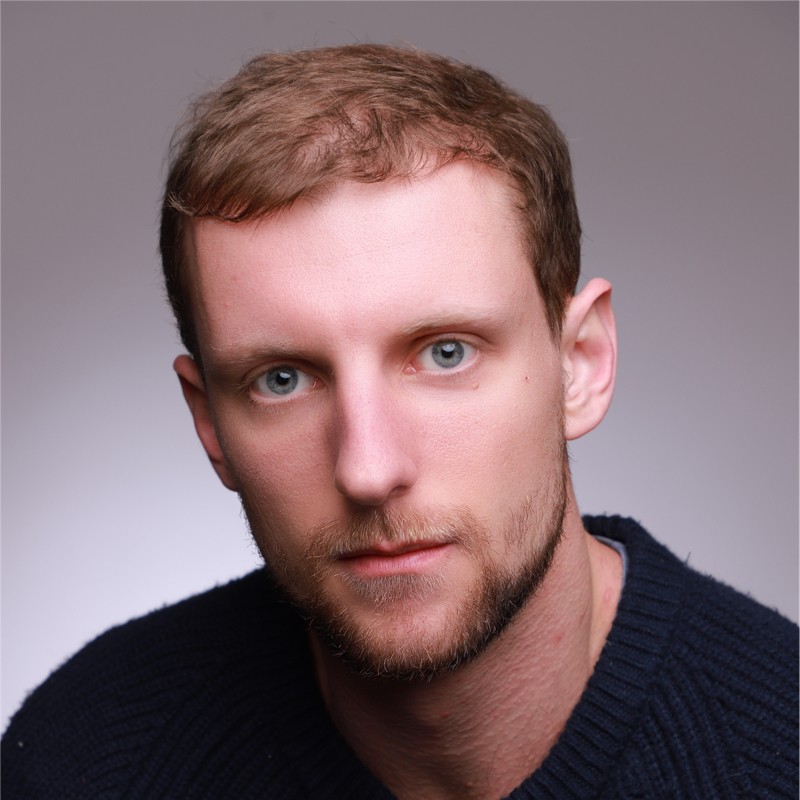
Saturnin Pugnet
founding member, tools for humanity
"New language models keep on coming; and techniques like fine-tuning, RAG, and GraphRAG add near-endless possibilities for literally each GenAI application call. Unify.ai simplifies and automates an optimization problem that is both daunting and universal, giving AI engineers visibility and control over cost, accuracy, and speed."
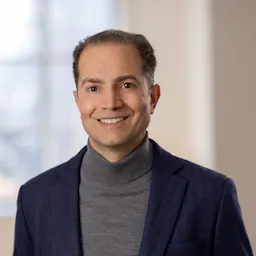
Philip Rathle
CTO at Neo4j
"One of the biggest challenges enterprise developers face in building production LLM applications (whether it's RAG or a complex agent workflow) is figuring out how to optimize it for better accuracy, cost, and speed. There are too many parameters to tune and it quickly becomes an intractable mess of trying out different hyperparameter values. Unify significantly reduces that complexity by optimizing the LLM selection, letting developers spend more time on critical application logic."

Jerry Liu
Ceo at LlamaIndex
"Trying to keep up with all new LLMs and providers is impossible, Unify makes it easy to cut through all the noise and ensure the best LLM is always being used."